Algebraic graph theory is the branch of mathematics that studies graphs by using algebraic properties of associated matrices. More in particular, spectral graph the-ory studies the relation between graph properties and the spectrum of the adjacency matrix or Laplace matrix. And the theory of association schemes and coherent con.
Spectral Graph Theory studies graphs using associated matrices such as the adjacency matrix and graph Laplacian. Let (G(V, E)) be a graph. We’ll let (n = |V|) denote the number of vertices/nodes, and (m = |E|) denote the number of edges. We’ll assume that vertices are indexed by (0,dots,n-1), and edges are indexed by (0,dots,m-1).
The aim of this book is to understand the spectral grpah theory. We combine all classic sources, e.g. Fan Chung’s book, Dan Spielman and Luca Trevisan’s graduate courses. Also some other important sources. There is not much to say in the introduction. If later I have some insightful ideas about this. Spectral graph theory is the study of properties of the Laplacian matrix or adjacency matrix associated with a graph. In this paper, we focus on the connection between the eigenvalues of the Laplacian matrix and graph connectivity. Also, we use the adjacency matrix of a graph to count the number of simple paths of length up to 3. Spectral Graph Theory and its Applications Yi-Hsuan Lin Abstract This notes were given in a series of lectures by Prof. Fan Chung in National Taiwan University. 1 Introduction 1.1 Basic notations Let G= (V;E) be a graph, where V is a vertex set and Eis an edge set. (Graph 1) We denote the edge set E= ffa;bg;fb;cg;g.
The adjacency matrix(A) is a (ntimes n) matrix with (A_{i,j} = 1) if ((i,j) in E) is an edge, and (A_{i,j} = 0) if ((i,j) notin E). If (G) is an undirected graph, then (A) is symmetric. If (G) is directed, then (A) need not be symmetric.
The degree of a node (i), (deg(i)) is the number of neighbors of (i), meaning the number of edges which (i) participates in. You can calculate the vector of degrees (a vector (d) of length (n), where (d_i = deg(i))), using matrix-vector mulpilication:begin{equation}d = A 1end{equation}where (1) is the vector containing all 1s of length (n). You could also just sum the row entries of (A). We will also use (D = diag(d)) - a diagonal matrix with (D_{i,i} = d_i).
The incidence matrix(B) is a (n times m) matrix which encodes how edges and vertices are related. Let (e_k = (i,j)) be an edge. Then the (k)-th column of (B) is all zeros except (B_{i,k} = -1), and (B_{j,k} = +1) (for undirected graphs, it doesn’t matter which of (B_{i,k}) and (B_{j,k}) is (+1) and which is (-1) as long as they have opposite signs).Note that (B^T) acts as a sort of difference operator on functions of vertices, meaning (B^T f) is a vector of length (m) which encodes the difference in fuction value over each edge.
You can check that (B^T 1_C = 0), where (1_C) is a connected component indicator ((1_C[i] = 1) if (i in C), and (1_C[i] = 0) otherwise). (Csubseteq V) is a connected component of the graph if all vertices in (C) have a path between them, and there are no vertices in (V) that are connected to (C) which are not in (C). This implies (B^T 1 = 0).


The graph laplacian(L) is an (n times n) matrix (L = D- A = B B^T). If the graph lies on a regular grid, then (L = -Delta) up to scaling by a finite difference width (h^2), but the graph laplacian is defined for all graphs.
Note that the nullspace of (L) is the same as the nullspace of (B^T) (the span of indicators on connected components).
In most cases, it makes sense to store all these matrices in sparse format.
Exercise¶
Lectures On Spectral Graph Theory
For an undirected graph (G(V, E)), let (n = |V|) and (m = |E|). Give an expression for the number of non-zeros in each of (A), (B), and (L) in terms of (n) and (m).
(A) and (B) both have (2m) non-zeros. (L) has (n + 2m) non-zeros.
Random Walks on Graphs¶
In a random walk on a graph, we consider an agent who starts at a vertex (i), and then will chose a random neighbor of (i) and “walk” along the connecting edge. Typically, we will consider taking a walk where a neighbor is chosen uniformly at random (i.e. with probability (1/d_i)). We’ll assume that every vertex of the graph has at least one neighbor so (D^{-1}) makes sense.
This defines a Markov Chain with transition matrix (P = A D^{-1}) (columns are scaled to 1). Note that even if (A) is symmetric (for undirected graphs) that (P) need not be symmetric because of the scaling by (D^{-1}).
The stationary distribution (x) of the random walk is the top eigenvector of (P), is guaranteed to have eigenvalue (1), and is guaranteed to have non-negative entries. If we scale (x) so (|x|_1 = 1), The entry (x_i) can be interpreted as the probability that a random walker which has walked for a very large number of steps is at vertex (i).
Page Rank¶
PageRank is an early algorithm that was used to rank websites for search engines. The internet can be viewed as a directed graph of websites where there is a directed edge ((i, j)) if webpage (j) links to webpage (i). In this case, we compute the degree vector (d) using the out-degree (counting the number of links out of a webpage). Then the transition matrix (P = A D^{-1}) on the directed adjacency matrix defines a random walk on webpages where a user randomly clicks links to get from webpage to webpage. The idea is that more authoritative websites will have more links to them, so a random web surfer will be more likely to end up with them.
One of the issues with this model is that it is easy for a random walker to get “stuck” at a webpage with no out-going links. The idea of PageRank is to add a probability (alpha) that a web surfer will randomly go to another webpage which is not linked to by their current page. In this case, we can write the transition matrixbegin{equation}P = (1-alpha) A D^{-1} + frac{alpha}{n} 11^Tend{equation}We then calculate the stationary vector (x) of this matrix. Websites with a larger entry in (x_i) are deemed more authoritative.
Note that because (A) is sparse, you’ll typically want to encode (frac{1}{n}11^T) as a linear operator (this takes the average of a vector, and broadcasts it to the appropriate shape). For internet-sized graphs this is a necessity.
Let’s look at the Les Miserables graph, which encodes interactions between characters in the novel Les Miserables by Victor Hugo.
Let’s now construct the PageRank matrix and compute the top eigenpairs
The Graph Laplacian¶
Spectral Embeddings¶
Spectral embeddings are one way of obtaining locations of vertices of a graph for visualization. One way is to pretend that all edges are Hooke’s law springs, and to minimize the potential energy of a configuration of vertex locations subject to the constraint that we can’t have all points in the same location.
Spectral Graph Theory Book Review
In one dimension:begin{equation}mathop{mathsf{minimize}}x sum{(i,j) in E} (x_i - x_j)^2text{subject to } x^T 1 = 0, |x|_2 = 1end{equation}
Note that the objective function is a quadratic form on the embedding vector (x):begin{equation}sum_{(i,j)in E} (x_i - x_j)^2 = x^T B B^T x = x^T L xend{equation}
Because the vector (1) is in the nullspace of (L), this is equivalent to finding the eigenvector with second-smallest eigenvalue.
For a higher-dimensional embedding, we can use the eigenvectors for the next-largest eigenvalues.
Attention: the first formula is not shown in the current notebook!
Spectral Clustering¶
Spectral clustering refers to using a spectral embedding to cluster nodes in a graph. Let (A, B subset V) with (A cap B = emptyset). We will denotebegin{equation}E(A, B) = {(i,j) in E mid iin A, jin B}end{equation}
One way to try to find clusters is to attempt to find a set of nodes (S subset V) with (bar{S} = V setminus S), so that we minimize the cut objectivebegin{equation}C(S) = frac{|E(S, bar{S})|}{min {|S|, |bar{S}|}}end{equation}
The Cheeger inequality bounds the second-smallest eigenvalue of (L) in terms of the optimal value of (C(S)). In fact, the way to construct a partition of the graph which is close to the optimal clustering minimizing (C(S)) is to look at the eigenvector (x) associated with the second smallest eigenvalue, and let (S = {i in V mid x_i < 0}).
As an example, let’s look at a graph generated by a stochastic block model with two clusters. The “ground-truth” clusters are the ground-truth communities in the model.
Now, let’s use spectral clustering to partition into two clusters
We’ll use the adjusted rand index to measure the quality of the clustering we obtained. A value of 1 means that we found the true clusters.
In general, you should use a dimension (d) embedding when looking for (d+1) clusters (so we used a dimension 1 embedding for 2 clusters). Let’s look at 4 clusters in a SBM
we’ll use K-means clustering in scikit learn to assign clusters.
Exercise¶
We’ll consider the stochastic block model with (k=5) clusters and (n=25) nodes per cluster.
Let (p) be denote the probability of an edge between nodes in the same cluster, and (q) denote the probability of an edge between nodes in different clusters.
Plot a phase diagram of the adjusted rand index (ARI) score as (p) and (q) both vary in the range ([0,1])
Introduction
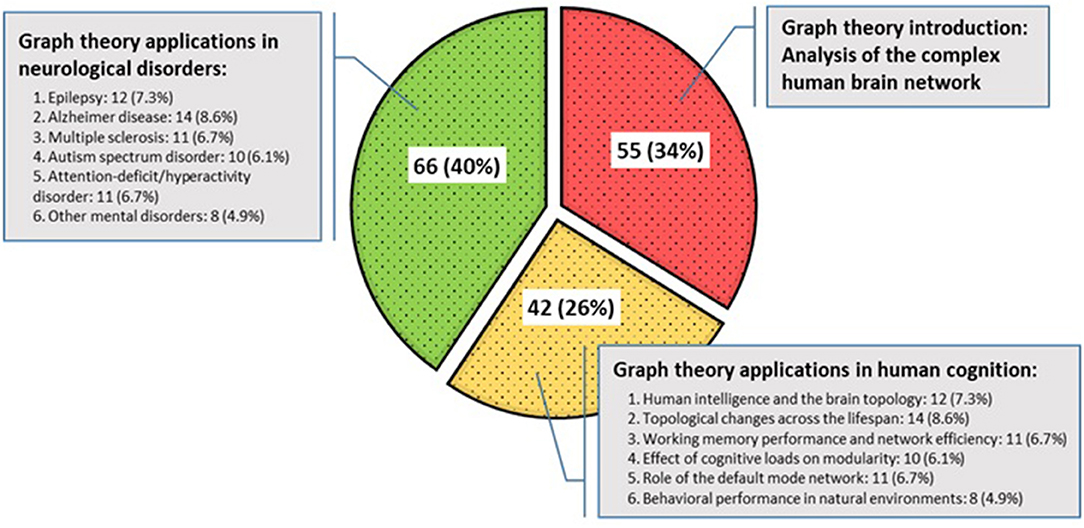
Spectral graph theory looks at the connection between the eigenvalues of a matrix associated with a graph and the corresponding structures of a graph. The four most common matrices that have been studied for simple graphs (i.e., undirected and unweighted edges) are defined by associating the vertices with the rows/columns as follows.
- The adjacency matrixA which is defined by placing a 1 in an entry in which there is an edge and a 0 otherwise.
- The (combinatorial) LaplacianL:=D-A where D is the diagonal matrix of degrees and A is the adjacency matrix.
- The signless LaplacianQ:=D+A where D is the diagonal matrix of degrees and A is the adjacency matrix.
- The normalized Laplacian
L:=D-1/2LD-1/2 where D is the diagonal matrix of degrees and L is the combinatorial Laplacian. By convention when a vertex has degree 0 (i.e., is isolated) then the corresponding entry of D-1/2 is 0.
There are many simple properties of graphs that can be deduced from the eigenvalues of the matrices. For example, the number of edges (via the adjacency, Laplacian and signless Laplacian), the number of connected components (via the adjacency, Laplacian and normalized Laplacian), being bipartite (via the adjacency and normalized Laplacian), and the number of bipartite components (via the signless Laplacian). For many people this is the extent to which they see the eigenvalues of matrices applied to graphs, and while these are interesting graph parameters to measure they do not show the strength of spectral graph theory.

We have gathered here a collection of various applications that have been developed that use spectral graph theory, sometimes in a surprising and unexpected way, to the study of graphs. This list is not meant to be exhaustive, but rather to give a taste for what is possible to do with the eigenvalues of a graph.
Useful surveys
More information about spectral graph theory and some of the applications in the wild, can be found in several monologues and surveys.
- Spectra of graphs -- a survey of spectral graph theory written by Andries Brouwer and Willem Haemers. This is one of the best places to start learning about spectral graph theory.
- Some applications of the Laplace eigenvalues of graphs -- a survey of applications of the combinatorial Laplacian written by Bojan Mohar, fairly comprehensive on what can be done with the Laplacian.
- Eigenvalues in combinatorial optimization -- a survey written by Bojan Mohar and Svatopluk Poljak.
- Spectral theory of graphs based on the signless Laplacian -- a survey of the signless Laplacian written by Dragos Cvetkovic. This contains material from several surveys about the signless Laplacian and is a good introduction to why it is an interesting matrix and how it connects to other matrices.
- Spectral graph theory -- a book focused on the definition and development of the normalized Laplacian written by Fan Chung, the first four chapters of the revised version are available online. This is the classic book for the normalized Laplacian.
- Spectral graph theory and its applications -- a class website for a course taught at Yale by Dan Spielman. Includes several lecture notes with some applications.
- Graph spectra in computer science -- a set of slides prepared by Dragos Cvetkovic giving an overview of applications of spectral graph theory to computer science, includes useful references and pointers to other papers. Also the paper version has appeared written by Dragos Cvetkovic and Slobodan Simic.
- The slides includes the following quote: 'Our general suggestion is that mathematicians should react on the explosion of the number of papers in computer science which use graph spectra by selecting for their own research some subjects from or inspired by such applications.'
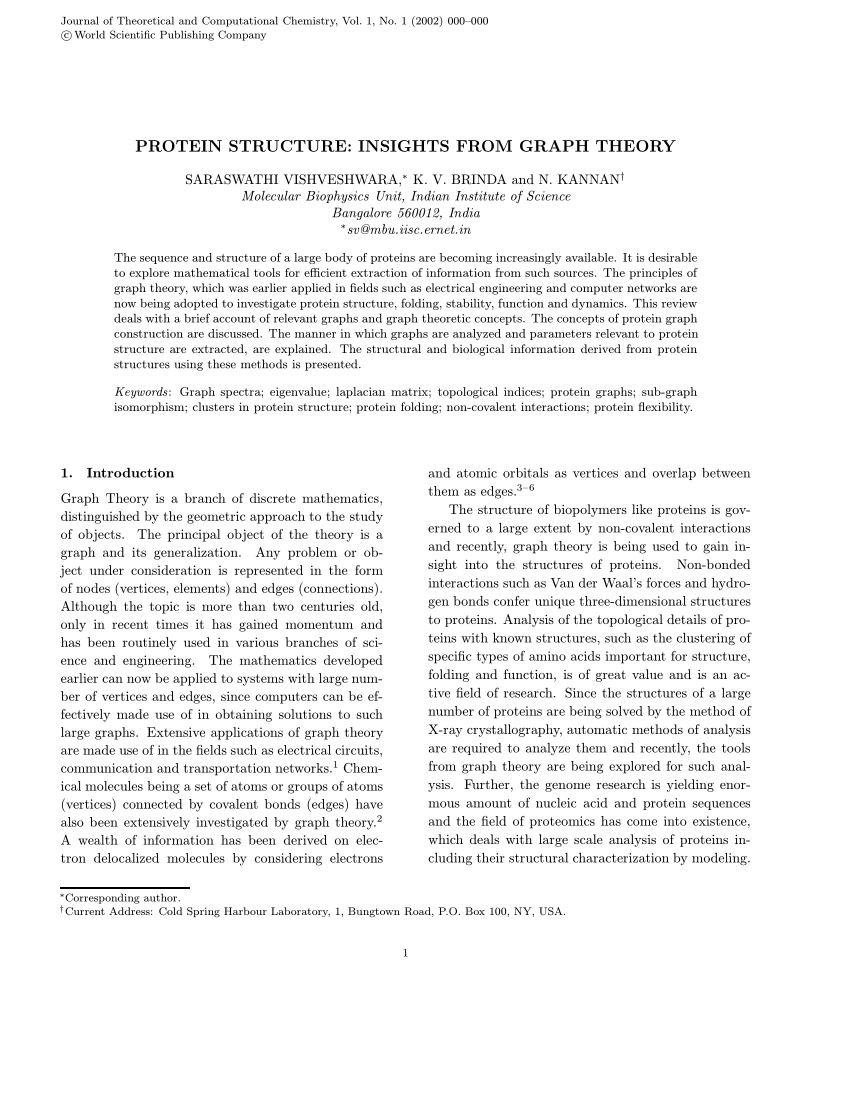
Applications of eigenvalues
- Showing that two graphs are non-isomorphic. If two graphs have differing sets of eigenvalues for some matrix then the graphs cannot be isomorphic (i.e., same up to relabeling). As a result many papers in spectral graph theory deal with deciding what eigenvalues can and cannot distinguish and showing that certain graphs are uniquely determined by their eigenvalues. A major problem is that many graphs have cospectral mates and so cannot be determined by their spectrum.
- Hoffman bound for the chromatic number, and various extensions. (See, for example, the paper Tales of Hoffman: Three extensions of Hoffman's bound on the graph chromatic number by Yonatan Bilu for some extensions. The original bound is classical and can be found in the survey by Andries Brouwer and Willem Haemers.)
- Finding small Folkman graphs, i.e., graphs with no K4 but any 2-coloring contains a monochromatic K3. (See the paper Explicit construction of small folkman graphs by Lincoln Lu.)
- Graham-Pollak Theorem which shows that Kn cannot be decomposed into fewer than n-1 complete bipartite graphs. (There are many proofs of this classical result, curiously they almost all rely on linear algebra in some way.)
- Rate of convergence of random walks. This is closely tied to the normalized Laplacian, since the eigenvalues of the normalized Laplacian are the same as those of the probability transition matrix of a random walk. (This is a well studied topic. See, for example, chapter 1.5 in the book of Fan Chung.)
- Lower bounds for the size of matchings in graphs. (This has been well studied by Sebi Cioaba and coauthors. See, for example, Edge-connectivity, eigenvalues and matchings in regular graphs by Suil O and Sebastian Cioaba and Matchings in regular graphs from eigenvalues by Sebastian Cioaba, David Gregory and Willem Haemers.)
- Counting triangles in graphs, more generally counting the number of closed walks of length k by looking at the eigenvalues of the adjacency. Related to this is the paper Lower bounds on the number of triangles in a graph by David Fisher which uses eigenvalues of the complement of a graph to give a lower bound for a root of a certain polynomial which relates to density of complete graphs, in particular triangles.
- Lovasz theta function gives a method to bound the Shannon capacity of a graph. In general this is very hard, but some results are known for some graphs, such as C5 by using eigenvalue bounds. (This can be found in On the Shannon capacity of a graph by Laszlo Lovasz, also in Proofs from the book by Martin Aigner and Gunter Ziegler.)
- The graph K10 cannot be (edge) decomposed into a union of three copies of a Petersen graph. (The number of edges is not enough to rule this out, see the survey by Brouwers and Haemers for a proof.)
- Showing a graph is Hamiltonian. One approach is to show that a graph is quasi- or pseudo-random and then use properties about such graphs. This approach was used to great effect in Sparse pseudo-random graphs are Hamiltonian by Michael Krivelevich and Benny Sudakov (this was modified to show that one can drop the assumptions of regularity in Small spectral gap in the combinatorial Laplacian implies Hamiltonian by Steve Butler and Fan Chung). An alternative approach is to show that a graph has high minimum degrees. This was done in Spectral radius and Hamiltonicity of graphs by Miroslav Fiedler and Vladimir Nikiforov.
- Bounding discrepancy in graphs, a measurement of the maximum difference in the number of edges and the expected number of edges between subsets of a graph. For regular graphs this is a well known bound which was extended to general graphs by use of the normalized Laplacian in Using discrepancy to control singular values for nonnegative matrices by Steve Butler.
- Bounds for the Cheeger constant of a graph, a measurement of the smallest cut to subdivide the graph into two 'large' parts. See chapter 2.2 of the book by Fan Chung for more information.
- Showing that a graph must contain all short cycles. It is possible for a graph to have the largest eigenvalue of the adjacency matrix near n/2 and not contain a triangle (i.e., think of a complete bipartite graph). However in A spectral condition for odd cycles in graphs by Vladimir Nikiforov it is shown that once we go just a little over n/2 that not only must we have a triangle (for n large) but we have all cycles of length at most n/320.
- Constructing super concentrators and expanders. These graphs have many applications in communication networks, for example. See, for example, λ1, isoperimetric inequalities for graphs and superconcentrators by Noga Alon and Vitali Milman.
- Bounding the diameter of a graph. (This is a classical result based on the number of distinct eigenvalues of the adjacency matrix. In The diameter and Laplacian eigenvalues of directed graphs by Fan Chung this was extended to directed graphs.)
- Showing graphs are quasi-random. Quasi-random graph properties are a collection of properties of graphs such that if a graph satisfies one of the properties it must satisfy all of the remaining properties. For example, it can be shown that if all but the eigenvalues of the adjacency matrix are 'small' then the graph must contain approximately the right number of Petersen graphs (or any specified graph). See Quasi-random graphs by Fan Chung, Ron Graham and Richard Wilson for more information.
- Bounds on independence number. This has an interesting application for Erdos-Ko-Rado theorems in that we can reduce the problem of largest intersecting family of elements to a largest independent set in a graph (where each vertex is an element and there is an edge if and only if the two elements do not intersect). See, for example, the slides Erdos-Ko-Rado Theorems written by Chris Godsil (among many others).
- Interlacing eigenvalues can be used to show that a graph satisfies certain properties. For example in showing the second neighborhood of a (connected) strongly regular graph must be connected (this was discussed in the combinatorics seminar given by Sebi Ciaobo).
- Measuring toughness, a variant of connectivity. See, for example, the paper Spectrum and connectivity of graphs by Andries Brouwer
Applications of eigenvectors
- Ranking.
- Cutting.
- Drawing.
- Clustering.
- Searching.
- Bandwidth.
Site maintained by Steve Butler.
Please send any requests/complaints to butler@math.ucla.edu.
Changes to the site are currently allowed by anyone, as long as this use is not abused!
Comments are closed.